The Impact of Machine Learning on Fraud Detection in Real-Time
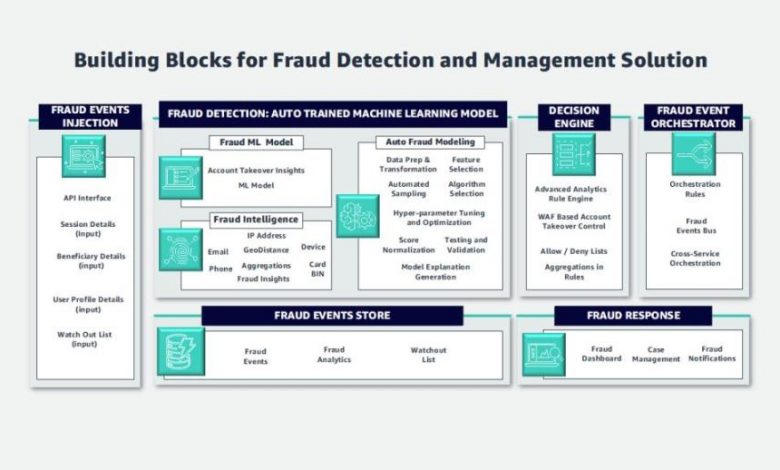
The Impact of Machine Learning on Fraud Detection in Real-Time
Introduction
Fraud is a pervasive problem in today’s digital world, and traditional methods of fraud detection are often not enough to keep up with the increasing complexity and sophistication of fraudulent activities. However, with the advent of machine learning, there has been a significant shift in the way fraud detection is approached.
The Role of Machine Learning in Fraud Detection
1. Real-time Analysis
Machine learning algorithms can analyze vast amounts of data in real-time, enabling businesses to detect fraud as it happens, rather than after the fact. This allows for faster responses and immediate action to prevent further damage.
2. Increased Accuracy
Machine learning algorithms are trained to detect patterns and anomalies in data, allowing for more accurate identification of fraudulent activities. By analyzing large datasets and learning from past incidents, these algorithms can constantly adapt and improve their detection capabilities.
3. Reduced False Positives
Traditional fraud detection methods often result in a high number of false positives, which can be time-consuming and costly to investigate. Machine learning can help minimize these false positives by accurately identifying genuine transactions and activities, saving businesses valuable time and resources.
4. Early Detection
Machine learning algorithms can detect subtle patterns and anomalies that may indicate the early stages of fraudulent activities. By identifying these early warning signs, businesses can take proactive measures to prevent fraud before it escalates and causes significant damage.
FAQs About Machine Learning in Fraud Detection
Q1. How does machine learning detect fraudulent activities?
Machine learning algorithms analyze large volumes of data to identify patterns and anomalies associated with fraudulent behavior. By continuously learning from new data, these algorithms can improve their detection accuracy over time.
Q2. Can machine learning algorithms adapt to new fraud techniques?
Yes, machine learning algorithms can adapt to new fraud techniques. As they analyze new data, they can learn and identify emerging patterns and behaviors associated with fraudulent activities. This adaptability makes machine learning an effective tool in combating evolving fraud tactics.
Q3. Does machine learning eliminate the need for human intervention in fraud detection?
While machine learning can automate and streamline the fraud detection process, human intervention is still crucial. Human professionals are needed to analyze the results generated by machine learning algorithms, perform investigations, and make decisions based on their expertise and judgment.
Q4. Are there any limitations to machine learning in fraud detection?
Machine learning in fraud detection is not without limitations. One limitation is the reliance on historical data for training. If fraud patterns change too rapidly, it may take time for machine learning algorithms to adapt and detect new techniques. Additionally, machine learning algorithms can also be vulnerable to adversarial attacks, where fraudsters attempt to manipulate the algorithms’ behavior.
Conclusion
Machine learning has revolutionized fraud detection in real-time, offering businesses a proactive and accurate approach to combating fraudulent activities. By leveraging the power of machine learning algorithms, organizations can effectively detect and prevent fraud, reducing financial losses and protecting their reputation. However, it is crucial to remember that machine learning is not a standalone solution and should be used in conjunction with human expertise to achieve the best possible results.